Notice
Recent Posts
Recent Comments
Link
일 | 월 | 화 | 수 | 목 | 금 | 토 |
---|---|---|---|---|---|---|
1 | 2 | 3 | 4 | 5 | ||
6 | 7 | 8 | 9 | 10 | 11 | 12 |
13 | 14 | 15 | 16 | 17 | 18 | 19 |
20 | 21 | 22 | 23 | 24 | 25 | 26 |
27 | 28 | 29 | 30 |
Tags
- 핀테크
- 전환율
- 그로스해킹
- 퍼널분석
- 한장으로끝내는비즈니스모델100
- 머신러닝
- retention
- 코호트
- sklearn
- 인게이지먼트
- 데이터분석
- pmf
- 리텐션
- mysql설치 #mysql #mysqluser #mysqlworkbench
- 바로팜
- activation
- 서말리포켓
- 셀프스토리지
- 올라
- BM분석
- 활성화
- fundbox
- allra
- 비즈니스모델
- 팔방이익구조
- model_selection
- CAC
- 역설구조
- 선정산서비스
- aarrr
Archives
- Today
- Total
데이터로그😎
[차원축소] LDA (Linear Descriminant Analysis) 본문
LDA vs PCA
PCA | LDA | |
특징 |
|
|
진행 과정 |
|
|
LDA 2차원 축소
from sklearn.discriminant_analysis import LinearDiscriminantAnalysis
from sklearn.preprocessing import StandardScaler
from sklearn.datasets import load_iris
iris = load_iris()
# 스케일링
iris_scaled = StandardScaler().fit_transform(iris.data)
# LDA
lda= LinearDiscriminantAnalysis(n_components = 2)
# LDA는 클래스의 정보를 같이 입력해줘야 한다.
# 지도학습처럼 feature, target이 같이 들어간다.
lda.fit(iris_scaled, iris.target)
iris_lda = lda.transform(iris_scaled)
import matplotlib.pyplot as plt
import seaborn as sns
import pandas as pd
iris_lda_df = pd.DataFrame(
iris_lda,
columns =['component_1', 'component_2'])
iris_lda_df['target'] = iris.target
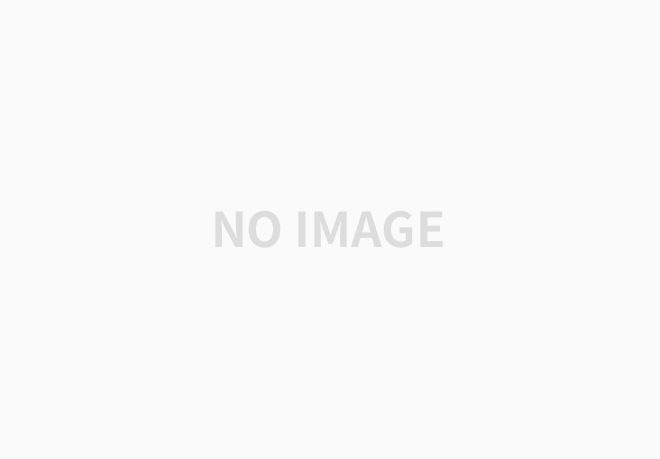
sns.scatterplot(
x='component_1',
y='component_2',
hue = 'target',
palette = 'muted',
data = iris_lda_df)
plt.title('LDA')
plt.show()
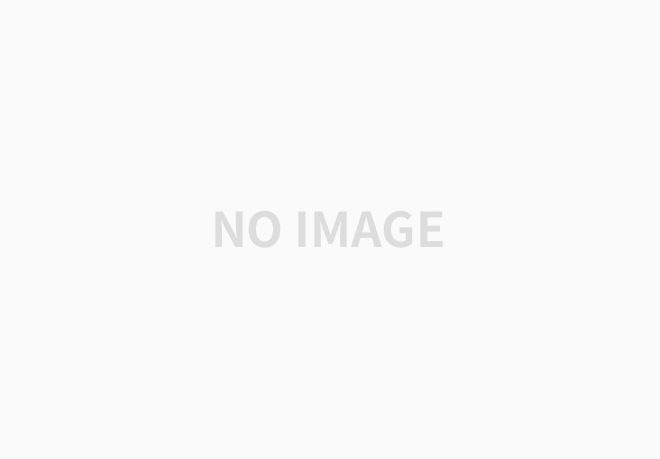
'#4. 기타 공부 > #4.2. 머신러닝' 카테고리의 다른 글
[비지도 학습] 군집 (clustering) (0) | 2023.09.05 |
---|---|
[차원축소] SVD (Singular Value Decomposition) (0) | 2023.09.05 |
[차원축소] PCA (Principal Component Analysis) (0) | 2023.09.05 |
[분류] 자전거대여 수요예측 (0) | 2023.09.05 |
[분류] 신용카드 사기 검출 (0) | 2023.09.04 |